
INTERDISCIPLINARY ARTIFICIAL INTELLIGENCE QUOTIENT SCALE

All industries are impacted by the digital transformation. With the digital revolution and Industry 4.0 come machine learning, big data and more. Outstanding user experience will be crucial, be it for your worker to faster understand new processes or a customer to order goods. Artificial Intelligence, personal assistants and other smart technologies are on the break of dawn. Does your car or fridge speak with you? Not yet, but in the foreseeable future they will and you will be thinking, how could I have ever lived without?
By defining the core principles to test AI systems and setting a standard for general artificial intelligence, you are able to understand AI and permanently improve it to enhance user experience. The core principles are defined interdisciplinary, allow measurement and comparability over time and can be updated without loosing old data and technical history.
AI will be the final and lasting competitive edge. But all well-meant intentions are ruined if it is not well implemented and continuously developed. Establishing and maintaining a headstart in your AI system is the key to long-term success.
The advantages of a widely accepted AI testing methodology at a glance:
-
Measure the status-quo
-
Compare to prior performance and competitors (benchmark)
-
Predict technical development and needs of users
-
Optimize and improve based on evidence


always
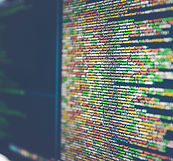
old data
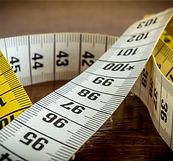
the future

always
Open Methodology
To understand artificial intelligence from an interdisciplinary perspective the huge corpus of psychological academic literature concerning human intelligence was confronted. Prerequisite and therefore the first step for the development of an A-IQ test is to derive a concept of intelligence. This framework is understood as a system of abilities to understand ideas (questions, commands for example) in a specific environment (e.g. put information into context) and to learn from experiences. This includes processing information as an experience (for example, something that has been learned beforehand) and engage in reasoning to solve problems (e.g. to answer questions or solve tasks).
The Interdisciplinary Artificial Intelligence Domains measure specific abilities based on recognized intelligence theories by Cattel & Horn, Kaufmann & Sternberg, as well as overlapping areas to the theories stated by Terman, Gardner and Guilford.
The framework integrates seven categories: Explicit Knowledge, Language Aptitude, Numerical and Verbal reasoning, Working Memory, Critical and Creative Thinking.
One Opportunity to Shape the Future

"Know What" in opposite to Know-How: Like information or data found in books or documents. Information which is readily available and can be transmitted to others through words, text, etc. Could also be called lexical knowledge and is similar to the concept of crystallized intelligence.
EXPLICIT KNOWLEDGE
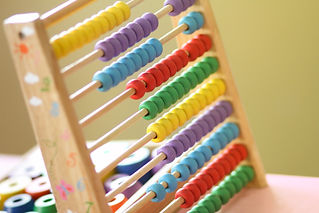
The ability to apply numerical facts to solve a stated problems in opposite to mathematical skills. It shows the ability to analyse and draw logical conclusions based on numerical data.
NUMERICAL REASONING

Creativity seen as a part of intelligence. Creativity operationalized as divergent thought process and the ability to solve problems or given tasks through generating multiple solutions. There is no correct answer, no one right answer, the answer is open ended and judged qualitatively, in opposite to convergent thinking where there is one right answer.
DIVERGENT THINKING
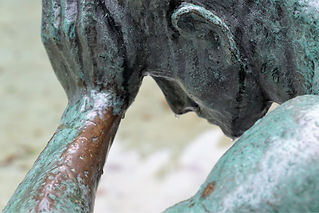
The ability to ask check-back-questions or simply put: to wonder. It show the ability to define and analyse a problem and to formulate counter questions adequately to get to a better solution. When critical thinking is applied pre-supposed answers (over-simplification) are avoided and various interpretations of the questions as well as answer-uncertainty is tolerated.
CRITICAL THINKING
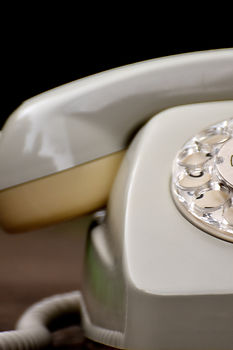
This category measures the ability to perceive or detect a language, to understand the content and answer the question in the same language. It measures the translation abilities for medium difficult sentences (not only single words, which is seen as a prerequisite) as well es the flexibility to switch between languages.
LANGUAGE APTITUDE

It is the ability to draw logical conclusions out of a given content. Concepts of the content are not framed in numbers but in words or concepts with a specific or ambiguous meaning.
VERBAL REASONING
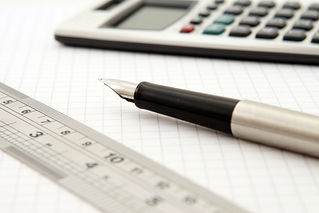
Working memory evaluates the ability hold information available for a certain amount of time for processing. This category evaluates the capacity to remember and retrieve random information within a few seconds, to repeat information and to answer questions based on prior conversation.
WORKING MEMORY
References
Knuth, D. 1972. The Art of Computer Programming, Volume 3: Sorting and Searching. 394-395.
Peter Stone, Rodney Brooks, Erik Brynjolfsson, Ryan Calo, Oren Etzioni, Greg Hager, Julia Hirschberg, Shivaram Kalyanakrishnan, Ece Kamar, Sarit Kraus, Kevin Leyton-Brown, David Parkes, William Press, AnnaLee Saxenian, Julie Shah, Milind Tambe, and Astro Teller. "Artificial Intelligence and Life in 2030." One Hundred Year Study on Artificial Intelligence: Report of the 2015-2016 Study Panel, Stanford University, Stanford, CA, September 2016. Doc: http://ai100.stanford.edu/2016-report. Ac-cessed: September 6, 2016.
Nils J. Nilsson, The Quest for Artificial Intelligence: A History of Ideas and Achievements (Cambridge, UK: Cambridge University Press, 2010).
Koch M., and Werther, S. 2013. Kreativität und Innovation in Organisationen – eine systemische Perspektive. Landes M., Steiner E. (eds) Psychologie der Wirtschaft. Psychologie für die berufliche Praxis. Springer VS, Wiesbaden
Zimbardo, P., Gerrig, R., and Graf, R. 2008. Psychologie. München: Pearson Education.
Kaufman, J. and Sternberg, R. 2010. The Cambridge handbook of creativity. New York: Cambridge University Press.
Validation of A-IQ Testing
IQ tests in the field of psychology measure intellectual abilities within a specific reference group: They rely on distinguishable components of general intelligence and are adapted to age, cultural background or people with mental or physical disabilities. Current IQ tests are standardized, which means that the individual result is compared to the mean score of the population. Renown IQ tests go under constant revision, to ensure that individual constructs are still validly measured. Comparison between tests or between different groups must be exercised with high caution and interpretations may not be applicable.
The A-IQ testing methodology was developed to overcome this limitation. Results between different products and test processing at different times should be comparable, because there will not be an infantile, adolescent and adult scale or parallel, different versions. This backward-comparability required some structural and mathematical optimization right from the start. It was required to establish a normalized weighting.
This introduced the possibility to improve and extend the questionnaire and still be able to compare to earlier versions. Adding new questions is very simple and can happen in a very modular way (without influencing or introducing complex dependencies). This is done with a certain level of an audit trail which allows solid tracking of changes.
The continuous extension and development of a large pool of questions, is critical to keep up with the latest developments in the fast pacing field of AI. But it is also important because the social and psychological perception of such solutions is also changing very fast. The requirements, usage and demands of users are changing with every iteration of new products.
The questionnaire will be evaluated on a regular basis to reflect these growing and reshaping demands. This will result in a new version of the questionnaire which will be available for analysts.
As the A-IQ testing methodology gains popularity, vendors of AI products will try to adapt the questionnaire to "emulate" understanding, which leads to higher testing scores although the products do not really exhibit intelligent behavior. A clear boundary between learning and understanding must be adhered to invariably.
To prevent this cheating effect a "dynamic test generation" is proposed where sentence structure and data points vary (e.g. "What is 2 times 3?” → "Calculate 3 times 2"). Punctual improvement and enhancement of the questionnaire will increase overall quality of the testing methodology.